Perspectives on estimation and modelling for large engineering projects
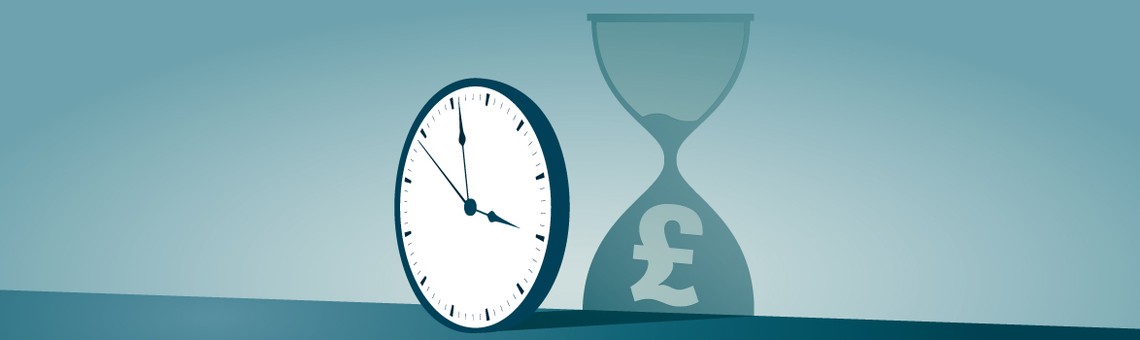
In a recent survey of over 160 Upstream 'megaprojects' (exceeding $1 Billion), with an average project size of $6.6 billion, EY1 identified 65% of projects as experiencing cost over-runs, and 78% experienced delays.
Perhaps this fact isn’t surprising; large CAPEX projects are inherently uncertain. Then again project costs, budgets and timescales can be modelled, and if realistic project estimates can be made then shouldn’t about 50% of projects be completed late, 50% early, 50% over budget, and 50% under budget? This wasn’t the case so we ask, what are the reasons for the cost over-runs and delays?
"Rather than talking about 'risk', with its negative implications, it may be better to look at 'variation' in either direction from a realistic base case or scenario, as uncertainty gives rise to opportunity as well as risk."
Perhaps the combination of key events and factors just played out worse than expected, and these factors had a similarly negative impact on most projects. The most important external factors identified include regulatory challenges, policy uncertainty, geopolitical uncertainty, and natural hazards.
However, we may find an alternative explanation if we consider some of the internal factors relating to project estimation and modelling.
Uncertainty
When making final investment decisions, the focus of attention is likely to be on the 'most likely', P50 or base case scenario. In practice, the actual outcome of a project is unlikely to be the base case.
One problem may be the existence of relatively rare events ('black swans') which we know may happen during the implementation of a major project. Such events lie in the 'tails' of the probability distribution used to estimate the likely cost and time outcomes of a project.
Rather than talking about 'risk', with its negative implications, it may be better to look at 'variation' in either direction from a realistic base case or scenario, as uncertainty gives rise to opportunity as well as risk. We need to find the best possible understanding of variation which is available, at proportionate cost.
Each decision criterion, such as Net Present Value, can be modelled as three components; the best estimate, and the positive and negative 'Tail Value at Risk', representing the change in risk profile which will arise from the decision.
Optimism and overconfidence
Optimism can lead to an over-favourable estimation of a P50 type value (median, but maybe also mean or 'most likely' mode). Over-confidence can lead to too narrow an estimation of variation; incorrect tails and underestimating risk. Put these together and you get disappointing outcomes more often than should be expected in stochastic modelling.
It is human nature to want to be optimistic; we want to undertake the project, and we want to get sanction. Studies suggest almost everyone is over-confident, especially experts answering questions in their own field. One possible remedy is 'calibration' of experts; everyone can attempt to calibrate themselves to see how over-confident they are and, by practice, attempt to correct this.
Model risk
Model risk is the adverse consequences arising from decisions based on incorrect models, or misused model outputs and reports. It is a quantitative risk, but hard to quantify.
- the model has fundamental errors and produces inaccurate or misleading or inaccurate results
- the model may be used incorrectly or inappropriately
Unfortunately, model risk has attributes which make it difficult to manage and it cannot be eliminated entirely. Some aspects and biases are subjective and cultural.
Difficulties arise in mapping model errors to actual financial impact; this lack of 'availability heuristic' (a bias) may be a reason for underestimating the impact.
A chosen probability distribution, often involves making implicit assumptions as well as explicit ones; using a uniform distribution to avoid modelling more knowledge than you actually have is a case in point. We recommend documenting the rationale underlying any estimate or expert judgement as thoroughly as possible, for ease of audit and review.
1Spotlight on oil and gas megaprojects, Oil and Gas Capital Projects series, EY 2014