Triangle-Free Reserving
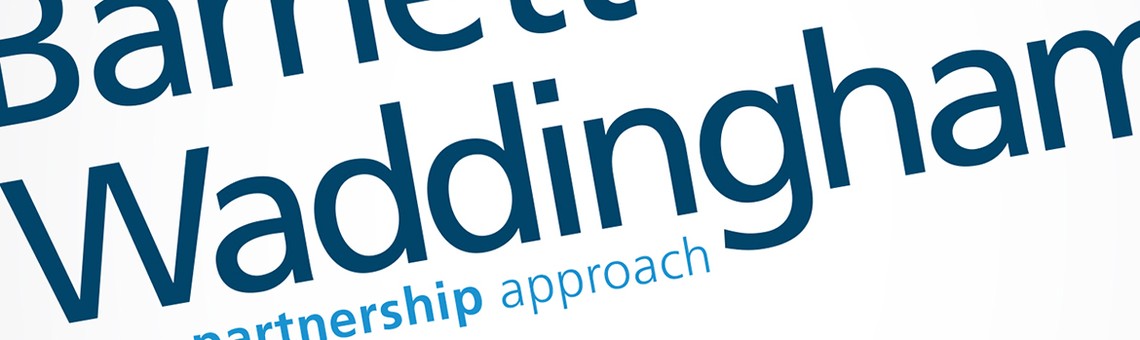
Jeremy Law contributed to the writing of this blog post
Pietro Parodi’s paper, Triangle-Free Reserving, has generated a great deal of interest among GI reserving actuaries. Pietro takes a critical look at some of the problems of currently popular reserving methods, and how they might be improved upon.
The paper won the Brian Hey prize for best paper submitted to 2012’s GIRO conference, and Pietro presented it again earlier this year at the Staple Inn. So what is all the fuss about?
It all starts with the triangle
A great deal of reserving work starts by summarising the available data into a claims triangle, grouping data into rows of origin years and columns of development years. By looking at the relationship between successive data points in the triangle we can make inferences along the lines of, 'If an underwriting year is now X years old, then we can expect the amount of claims incurred to increase by a factor of Y before it is completely run off'. Together with the current claims amounts, these relationships can be used to project an estimate for the ultimate claims amount, and therefore the reserves that should be held.
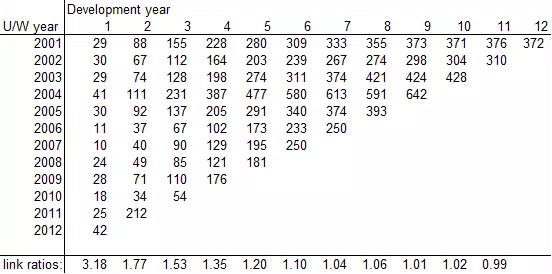
This approach and its many variants and extensions have proved successful and popular. When applied with due care and judgement, they give perfectly satisfactory point estimates of reserves. The basic chain ladder method also has an appealing elegance, with all the inputs and outputs clearly visible, and it gives a clear representation of how claims are expected to develop over time. Furthermore, the simplicity and widespread adoption of triangle-based methods make communicating and justifying their results comparatively straightforward.
The problem arises, Pietro argues, when you try to extend triangle methods beyond generating a point estimate to examine the distribution of reserves. There is a fundamental difficulty here because of how much data is lost in forming the triangle. Regardless of how much data you start off with, you end up with one data point per development period per origin period, which means you will hardly ever have enough information to get a real sense of the distribution. How can you expect to have a good idea of where the 99.5th percentile of reserves is if you restrict yourself to working from only 50 numbers?
There is a nice illustration of this point in the paper using a photography analogy: grouping data into a triangle is very much like compressing a digital image. In compressing an image, one loses information. No matter how cleverly you manipulate the compressed image afterwards, you cannot make out the fine detail (the number plates on distant cars, the time on a clock face, the 1 in 200 year events) if you only have a few smudgy pixels.
The argument then is that all triangle based reserving methods used to give a distribution of reserves are slightly spurious, and of especially limited value at the extreme ends of the distribution. Sadly the 'zoom and enhance' of Star Trek and CSI won’t work any better in an actuarial setting than it does on digital images!
Pietro puts forward an alternative framework for reserving. Like much of modern pricing work, this framework is based on the collective risk (i.e. frequency/severity) model. The comparison makes sense, as reserving can be thought of as retrospective pricing with a little more information available.
An example implementation is discussed in the paper. This uses a frequency model for the IBNR claims count based on the observed distribution of delays. Severities are then modelled, in this case using a kernel model where each year has its own severity distribution that is a scaled version of the kernel distribution, to account for claims inflation. Aggregate claims for each period are then simulated using Monte Carlo methods to give a distribution of IBNR, and UPR can be analysed along with these in exactly the same way. IBNER is dealt with separately (and assumed to be independent for the purposes of analysing the overall reserve distribution).
We’ll gloss most of the technical details for this blog, and instead show some results from an experiment Pietro did to compare this approach with the triangle-based Mack method. This experiment used artificially generated sets of claims data, where the 'true' IBNR distribution was known in advance and could be compared with the results from the two methods. This is shown in the following graph. The results from the triangle free method, represented with the blue triangles, closely match the 'true' IBNR distribution, represented by the red squares. Results from some other triangle based methods are shown for comparison, and these are less successful in replicating the distribution.
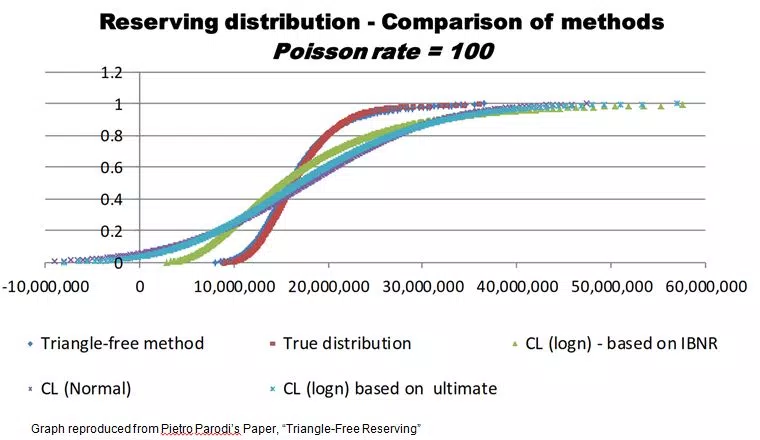
Therefore, the major advantage of this approach is that it may be possible to get a much better idea of the distribution of reserves than was possible using triangle based methods. This methodology doesn’t suffer the compression problem. It turns out that the answer to the question of how to pull off the 'zoom and enhance' trick is to not compress your image in step one!
So why might reserving practitioners be reluctant to abandon the trusted triangle?
The first difficulty is simply that the model is more complex. Increasing the model’s complexity means that it takes longer to build and work with. More expertise and training will be required, and ultimately, it makes carrying out the same work more expensive.
A second important point is that the method becomes harder to interpret and understand. People are rightly suspicious of 'Black Boxes'; an understanding of how the model comes up with the estimates that it does is essential if one is to understand (or apply judgement to) the results. As a model becomes more sophisticated there is a danger of losing sight of what is going on, and just blindly turning the handle to get an answer out. Also related to this is the problem of communicating results and justifying them, which gets harder as the model becomes more complex. However, dealing with complicated mathematical problems and communicating results from modelling them is very much the actuary’s job!
Finally, there are the practical considerations: the availability of computing power and data. The former is not too great a concern thanks to the relentless progress of Moore’s law and the power of modern computing hardware. The latter may be more of a sticking point! Deciding on frequency and severity distributions requires individual claims data to be available, rather than just the aggregated data that triangle methods require. It may be possible to make some reasonable assumptions, but nonetheless, data will sometimes be a limitation.
Ultimately, if one uses a more sophisticated and elaborate model, then one would hope to get a better answer. So in the end, this comes down to a core principal of all modelling that can be neatly summarised in an (apocryphal or otherwise) Einstein quote, “Everything should be made as simple as possible, but no simpler than that".