PPO stochastic models: Are they the bee’s knees?
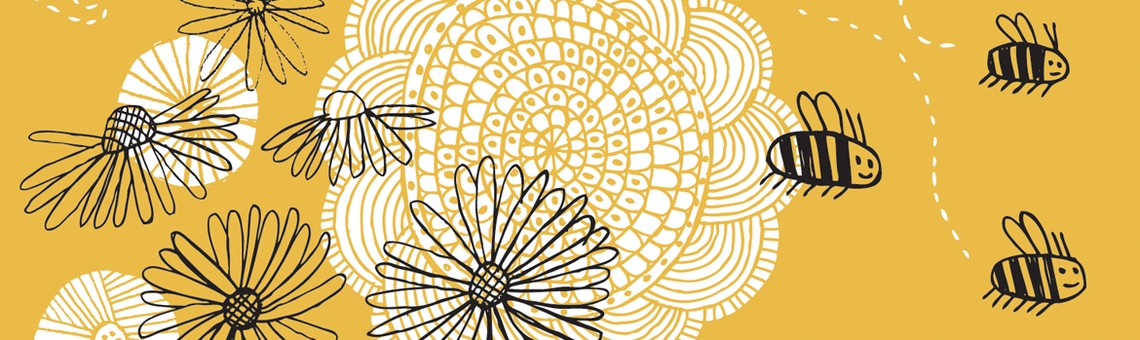
A stochastic model is a model where one or more of your inputs are random variables. Each time you run the model, the value of your random variables could differ. This means that each iteration of the model has the potential to yield different results.
A stochastic model is typically run many thousands of times and the results collated to form a probability distribution. Looking at the probability distribution of results gives you an idea of what the real life outcomes could be and how likely each one is. In contrast deterministic models only give you a point estimate and do not convey the fact that there are a whole range of possible outcomes, some of which may be more likely than other.
When using a stochastic model, it is important to know when it is and when it is not appropriate to model a parameter stochastically. The key considerations when deciding whether to make a parameter stochastic or not should be:
- How sensitive the model output is to that parameter – if the model is not that sensitive to the parameter in question, then it making the parameter stochastic will not add much value to your output.
- How much knowledge and understanding you have on what the parameter in question and its distribution should be – If you know or have a good idea of your uncertainty in the best estimate value of the parameter, then modelling it stochastically might be a good idea. If you have absolutely no idea about the uncertainty around your parameter, then you have to be careful of the risk of spurious accuracy arising from modelling it stochastically.
- How much will it increase your model runtime – stochastic models are computationally expensive and adding extra stochastic parameters will start to exponentially increase your model runtimes. Despite recent increases in computing power, there is still a cut-off point where the extra time the model takes to run will outweigh the benefits of having an extra stochastic parameter.
Periodic Payment Orders (PPOs)
PPOs are court awarded orders that are increasingly being used to settle personal injury claims. The order instructs the insurer to pay the claimant a regular amount, designed to cover the cost of care and compensate for loss of earnings resulting from the injury, for the rest of the claimant’s life.
They are a pain for general insurers as:
- There is limited historical data out there to analyse;
- There are huge uncertainties on the size of these liabilities;
- Some general insurers may not feel like they have all the necessary expertise to work with the long tailed mortality risk inherent in PPOs.
PPOs are very sensitive to the longevity risk inherent in them and so longevity risk is a good candidate to be modelled stochastically. Whilst there is very limited historical data on PPOs to set longevity assumptions, there is a lot of data and research available on the underlying shapes and patterns of mortality that you can combine with your historical experience to set your assumption and measure your uncertainty in it.
SAM
Barnett Waddingham has combined its technical expertise as well as their extensive knowledge of general and life insurance techniques to construct SAM. It is the Stochastic Analysis Model that we use to analyse portfolios of PPO liabilities.
SAM stochastically models three different aspects of longevity risk and it projects future PPO cashflows and calculates their total present value. Having this information will be useful to help with pricing future policies, reserving for current PPOs in payment and setting investment strategy.
SAM outputs
These are some example SAM outputs:
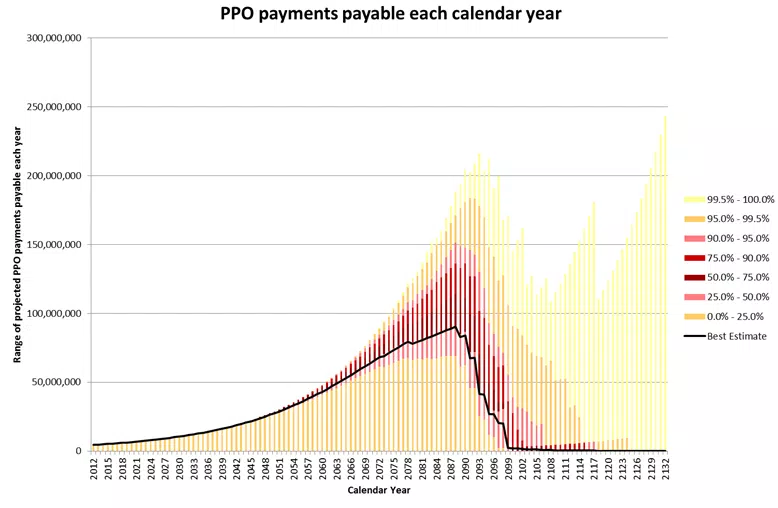
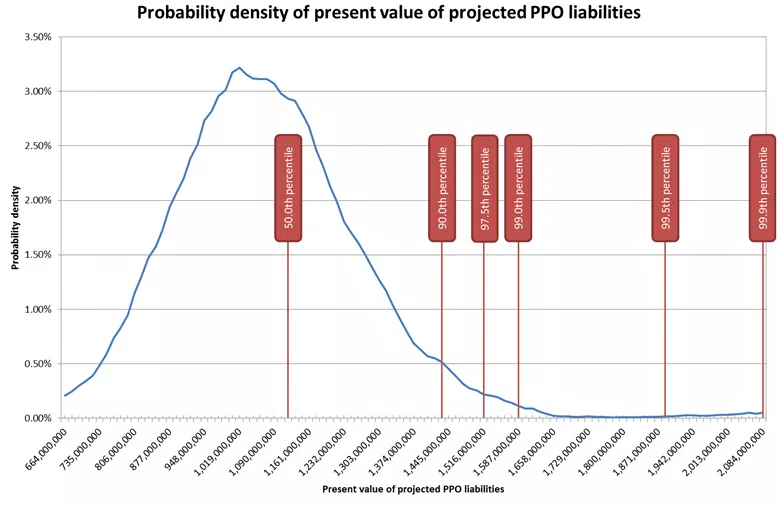
Honey, I know the future.
We believe that our stochastic model is in fact the bee’s knees when it comes to analysing PPO liabilities. Please contact us to discuss stochastic modelling or how we can use our model to help you