Data science, ethics and actuaries
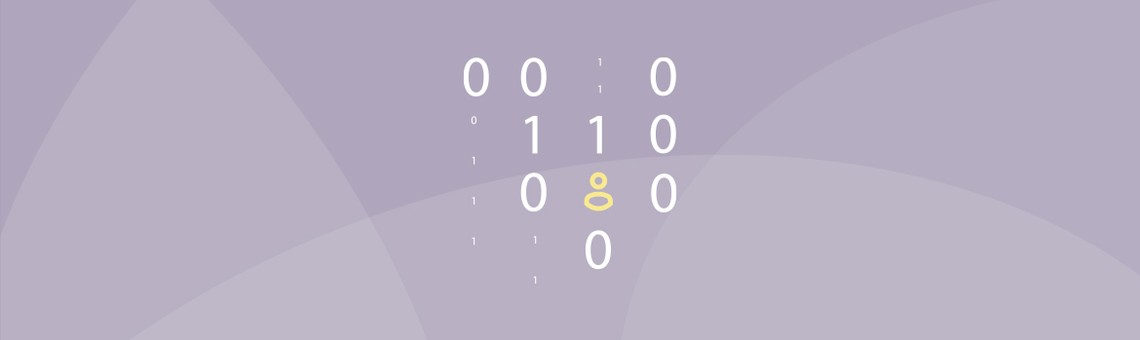
Estimated reading time: 3 minutes
There is no shortage of hype surrounding data science these days, particularly when it comes to the advances around machine learning and artificial intelligence (AI).
Given that data science brings together several fields – including maths, statistics and computer science – actuaries are often as excited about the possibilities of change promised by these technologies as the scientists themselves.
However, data science models are a double edged sword. Get it right and it can help you make better decisions and solve problems for society. Get it wrong and your company may face reputational and trust issues which have major commercial implications.
Where can data science go wrong?
Despite their impressive capabilities, machine learning/AI models are only as good as the data. The basic rule of 'garbage-in, garbage-out' still applies to these models. Therefore, as with any model, the risk of companies messing up in the name of data science is fairly high. We have previously discussed a few examples of how this has already been happening, including unconscious gender bias and transparency issues, in our previous data ethics blog.
What should actuaries consider about data ethics?
In order to help practitioners navigate the data science field better, the Institute and Faculty of Actuaries (IFoA) and Royal Statistical Society (RSS) have collaborated and published A Guide for Ethical Data Science. The guide talks about five themes and principles within data ethics:
- Seek to enhance the value of data science for society – understand the potential impact of the work and consider any opportunities that may deliver benefits for the public
- Avoid harm – beware of how data could potentially cause harm
- Apply and maintain professional competence – learn how to minimise uncertainty and risks by complying with best industry and professional practices and applying analytical rigour
- Seek to preserve or increase trustworthiness – build trust and an understanding of the work done with others
- Maintain accountability and oversight – agree who is accountable and the level of oversight that is put in place when delegating any decision-making to machines
This guide also gives some practical examples of how these principles could be put into practice. At the end of the guide there is a useful implementation checklist to help practitioners embed ethics into data science work. We urge you to read this guide if you are involved in any kind of modelling.
How is this guide relevant for practitioners?
The last three themes are very familiar to actuaries as these are drilled into them as part of their actuarial training. Traditionally, actuaries have been validating internal models for insurance companies to Solvency II validation standards. However, increasingly actuaries have been working in areas that involve understanding ethics and human behaviour; for example in pricing or climate change. This is where the first two themes will be important for practitioners to consider when doing more data science work.
As insurance companies are using more and more data science models, collaborations between actuaries and data scientists will be the key to developing a robust framework for building trustworthy ethical models.
Whether it’s using appropriate and responsibly sourced data, or avoiding unethical bias in models, actuaries have truly valuable lessons to share with data scientists and vice versa. Collaborating more could see us take huge leaps forward in positively transforming our society.
Further reading
Data democratisation: leveraging data to generate value
Insurance companies are collecting more and more data, in order to secure the best risks for the best price. So why are we still waiting for the 'big bang' to happen?
Find out moreStay up to date
Get the latest independent commentary and exclusive insights from a range of experts at the forefront of risk, pensions, investment and insurance – tailored to your preference.
Subscribe today